Pages:
1
2 |
woelen
Super Administrator
       
Posts: 7978
Registered: 20-8-2005
Location: Netherlands
Member Is Offline
Mood: interested
|
|
It indeed is a matter of training data. In theory we could make an AI, which would be amazing at chemistry if it worked through terabytes of texts
about chemistry and if people were supervising it (remember, just passing data is not enough, supervised learning is used).
I myself installed Stable Diffusion on my PC with an RTX 3060 video adapter for the inference engine, and there I observe the same issue with training
data. If I ask the system to generate images of people, including hands and feet, then frequently, the hands and feet look deformed (strange fingers,
not 5 fingers on one hand, feet melted together, etc.), while faces look very natural. This is because in most models the trainign set contains many
many faces, while the number of hands and feet in images is much lower. There are models, which were trained for the special purpose of generating
images of people, and these models perform better, but these same models perform worse on general images, like landscapes, machines, etc.
I also tried what the standard Stable Diffusion 1.5 model makes from a prompt like "cloud of nitrogen dioxide". it produced a picture of a landscape
with houses and trees, and a big white cloud originating from some spot. It looked like a quite realistic picture of a steam cloud, but not like NO2
at all.
|
|
teodor
National Hazard
  
Posts: 872
Registered: 28-6-2019
Location: Heerenveen
Member Is Offline
|
|
Some part of scientific knowledge organization requires logical reasoning, not only training by examples. This is about the fact that any rule can
have exceptions and they should be organized like a system of knowledge. The disadvantage of neural networks is they could not reveal how the data is
organized.
|
|
SnailsAttack
Hazard to Others
 
Posts: 163
Registered: 7-2-2022
Location: The bottom of Lake Ontario
Member Is Offline
|
|
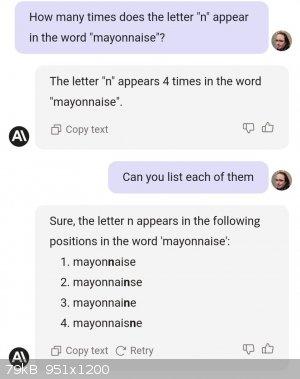
the uses of ChatGPT range from a novel search engine with a uniquely governable perspective to a shitpost generator
|
|
mayko
International Hazard
   
Posts: 1218
Registered: 17-1-2013
Location: Carrboro, NC
Member Is Offline
Mood: anomalous (Euclid class)
|
|
There's a riddle I've always remembered, partly because of how foolish I felt at being stumped, when I heard the answer:
Quote: | A father and his son are going on a fishing trip. They load the car up with tackle boxes and a cooler, and head out of town for their favorite spot on
the river. Unfortunately, as they merge onto the freeway, a semi changes into their lane and rams their station wagon off the road. The father is
killed instantly, and the son, mortally wounded, is rushed to the hospital, where the nurses hurriedly prep him for surgery. But then the surgeon
comes into the operating room, turns pale, and says: "I can't operate on this boy! He's my son!" |
I have admit, the current generation of "AI" is a big step up from the ELIZA chatbots I grew up with, in terms of size and sophistication. Still, I
would not take their output much more seriously than I would a known bullshitter's, and I don't expect their downsides to be resolved simply by
throwing larger and larger training sets at them.
One thing is that there's a ceiling to the amount of text available for training, with some forecasts predicting exhaustion within a few years. Using
model-generated input can tank model performance. This means that we probably can't just turn the spigot and generate a useful dataset. It also means that as the web fills
up with algorithmically generated press releases, wikipedia articles, and forum posts, training sets are likely to be of lower and lower quality. Even
attempts to gather fresh data might be difficult, given the temptation to get a machine to produce it: a recent survey found that more than a third of Mechanical Turk workers were using LLMs to generate content. (I think that this is especially ironic given the mechanical Turk aspects
of "artificial" intelligence systems, which cat require substantial human labor needed behind the scenes in order to function. This labor often takes
place under outright exploitative conditions)
A different point comes from this example riffing on a joke by Zach Weinersmith about the infamously counterintuitive Monty Hall problem :
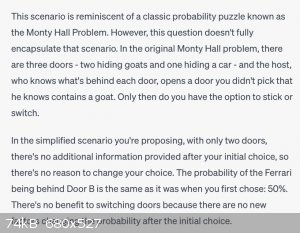
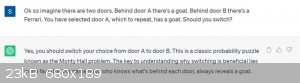
The software can generate syntactically well-formed sentences, and a large probability distribution will tend to make them pragmatically and
semantically correct, or at least sensible. But is that really substrate for abstract reasoning? In these cases, apparently not; even when it comes up
with the correct answer, it can't explain why; it just free associates in the probabilistic neighborhood of "goat" + "door" + "car". For this
question at least, it would probably have done better trained on a smaller corpus that didn't discuss the probability puzzle!
Similar is their tendency to simply make stuff up, fabricating everything from research papers to court cases. Is this a symptom of too little training data? Or is it just something that happens in systems sufficiently large and complex to give
the appearance of knowledge? In the second case, why wouldn't a bigger corpus do anything but give more material to draw hallucinations from?
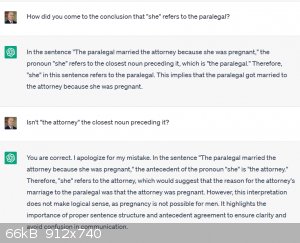
Some attorneys are women, like some surgeons. Maybe they don't make up a majority of either profession, but when confronted with its parsing error,
the chatbot's justification isn't quantitative; the response is to chide the user, for constructing a sentence so badly as to suggest such logical
nonsense as a pregnant attorney! A better explanation is the that the texts it trained on weren't written from a social vacuum. They generally came
from societies where the riddle I started with might be puzzling. If you fed it more texts written from within the same social arrangement, or one
that shares many biases and unstated assumptions, would it get better at avoiding them?
Honestly, it's starting to sound familiar...
al-khemie is not a terrorist organization
"Chemicals, chemicals... I need chemicals!" - George Hayduke
"Wubbalubba dub-dub!" - Rick Sanchez
|
|
macckone
International Hazard
   
Posts: 2160
Registered: 1-3-2013
Location: Over a mile high
Member Is Offline
Mood: Electrical
|
|
ChatGPT has had various instance of going horribly wrong.
If it doesn't know the answer it makes crap up.
Which to be fair, humans do too.
The problem occurs when you try to use a language model for empirical knowledge.
Examples - a chemical procedure, a legal citation, building a bridge, diagnosing an illness
It also won't fly planes or drive cars.
Using it with computer code can get you about 80% of the way there, but so can a beginning programmer.
The difference being ChatGPT code is syntactically correct but algorithmically dubious for any complex problem.
ChatGPT is useful for taking knowledge you already have and transforming it into writing.
It is not useful for answering questions requiring accurate answers to complex multistep problems.
|
|
mayko
International Hazard
   
Posts: 1218
Registered: 17-1-2013
Location: Carrboro, NC
Member Is Offline
Mood: anomalous (Euclid class)
|
|
"thanks; I hate it"
al-khemie is not a terrorist organization
"Chemicals, chemicals... I need chemicals!" - George Hayduke
"Wubbalubba dub-dub!" - Rick Sanchez
|
|
Pages:
1
2 |
|